In this post, you will learn how to interpret a heatmap for differential gene expression analysis. Find out why heatmaps are a great way of visualising gene expression data with this simple explanation.
Let’s dive in!
Prefer to listen? Watch my Youtube video on heatmaps for gene expression analysis by clicking on the video or here.
If you are more of a reader, find out how to interpret heatmaps in the sections below.
What is a heatmap?
A heatmap is just a type of plot. It is just one of the many ways we can visualise data. In the context of gene expression data, it is a great way to display the expression of many genes across many samples. Heatmaps allow us to find and visualise patterns in gene expression data.
A heatmap looks something like this:
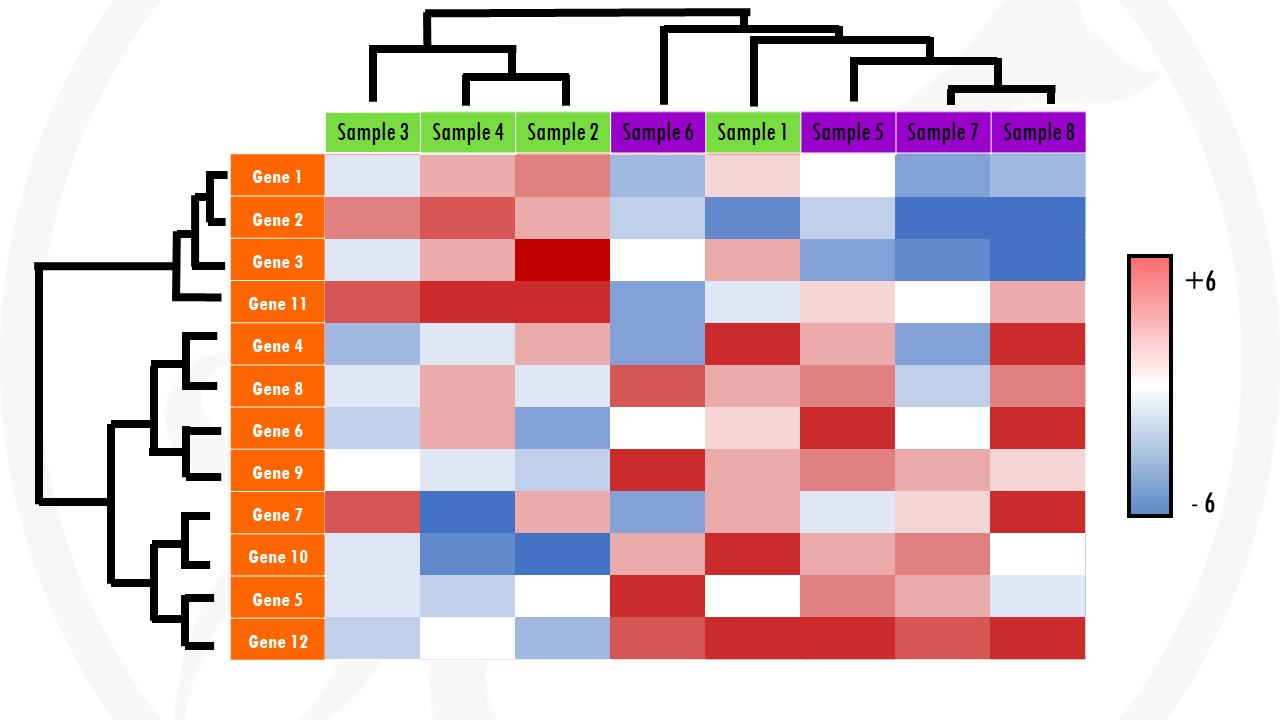
In a heatmap, each row usually represents a gene. Each column represents a sample.
What about the colours? The colour and intensity of the tiles or rectangles is used to represent changes (not absolute values) of gene expression. So, basically, instead of numbers, we use colours.
Let’s take a look at an example. Imagine you are comparing samples of cancer tissue and healthy tissue. You get gene expression data for many different genes from both tissues. The differential gene expression analysis results will tell you how much each gene is upregulated, or downregulated in the cancer samples compared to control samples. Our table would look something like this:
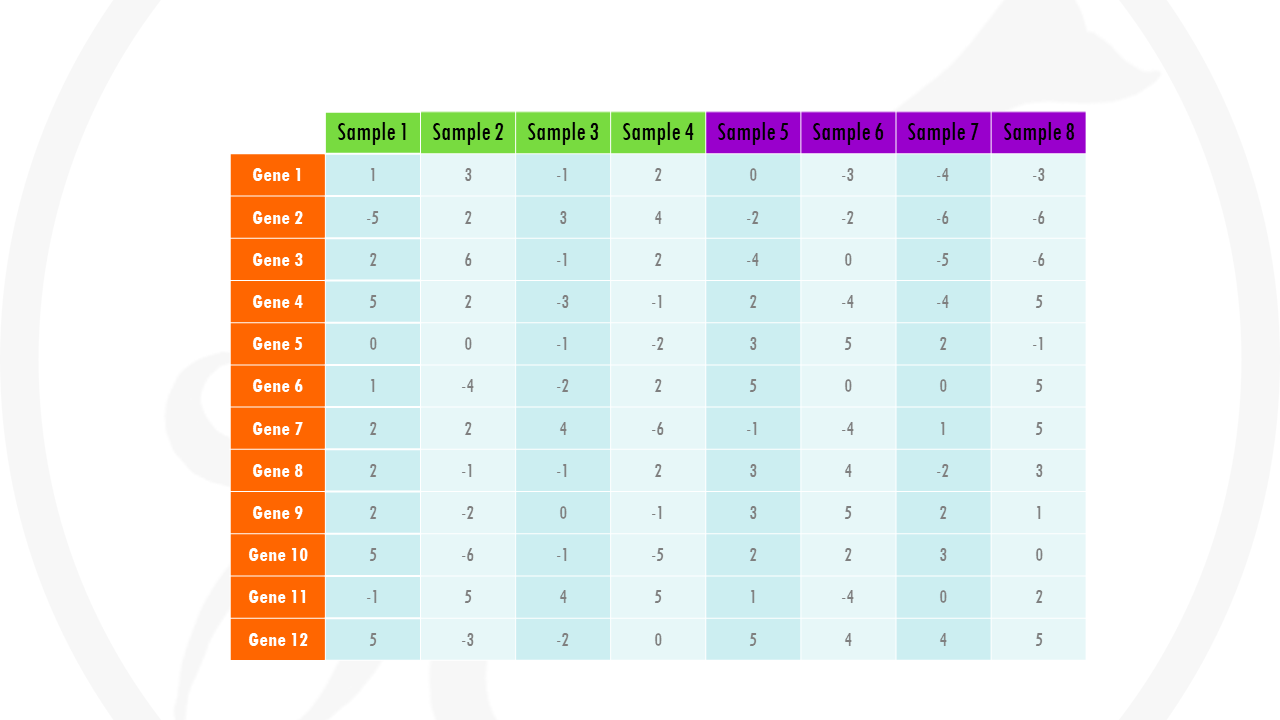
Instead of a table full of numbers, we can just convert them to a grid of colours. If our number is negative, we will give it a red colour. If our number is positive, we will give it a blue colour. The colour will be more intense the lower (or higher) the value is. If a gene does not change much between conditions (so it’s differential gene expression is close to 0), we will just give it a white colour.
And voila! We have our beautiful heatmap!
This way, we can easily visualise which genes are mostly upregulated, or downregulated, across samples.
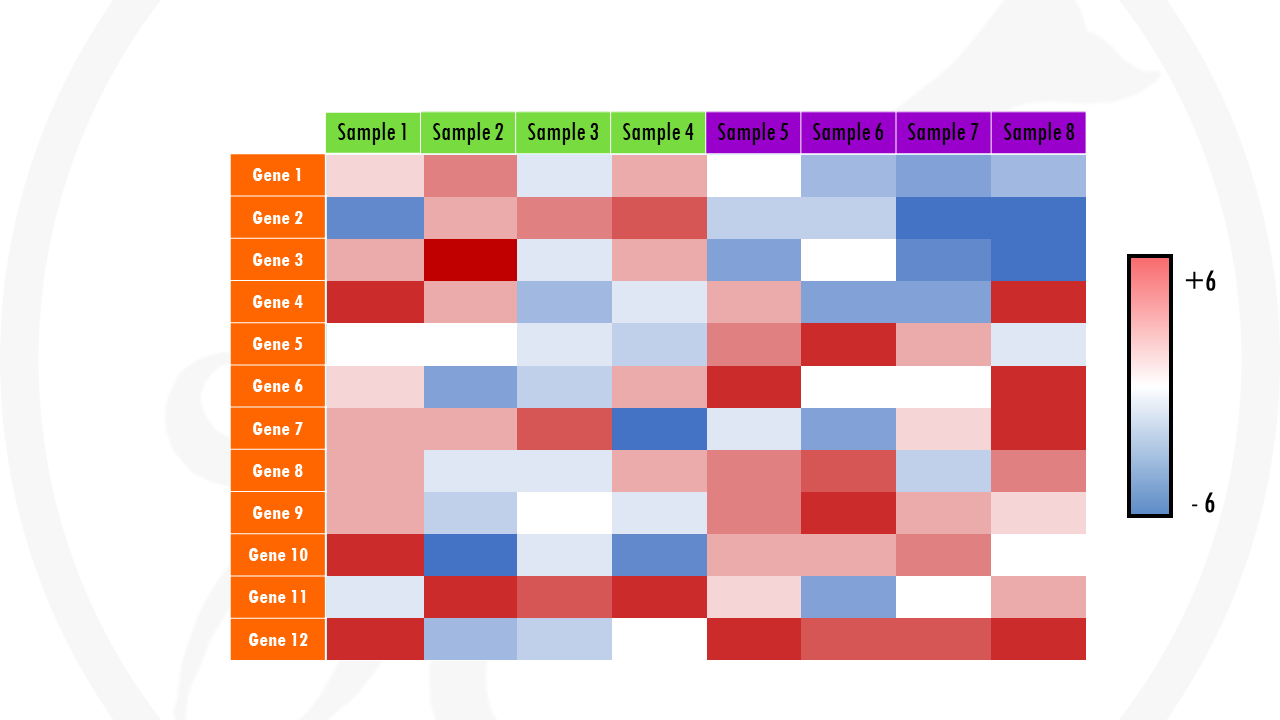
Squidtip
In a heatmap, gene expression values are depicted by colour. This way it is much easier to get a general overview of our gene expression data.
Clustered heatmaps
But, how can we see patterns with so many coloured tiles? The answer is to cluster the tiles. This involves a meaningful reordering of the rows and columns. And this is how we get a clustered heatmap. A clustered heatmaps looks something like this:
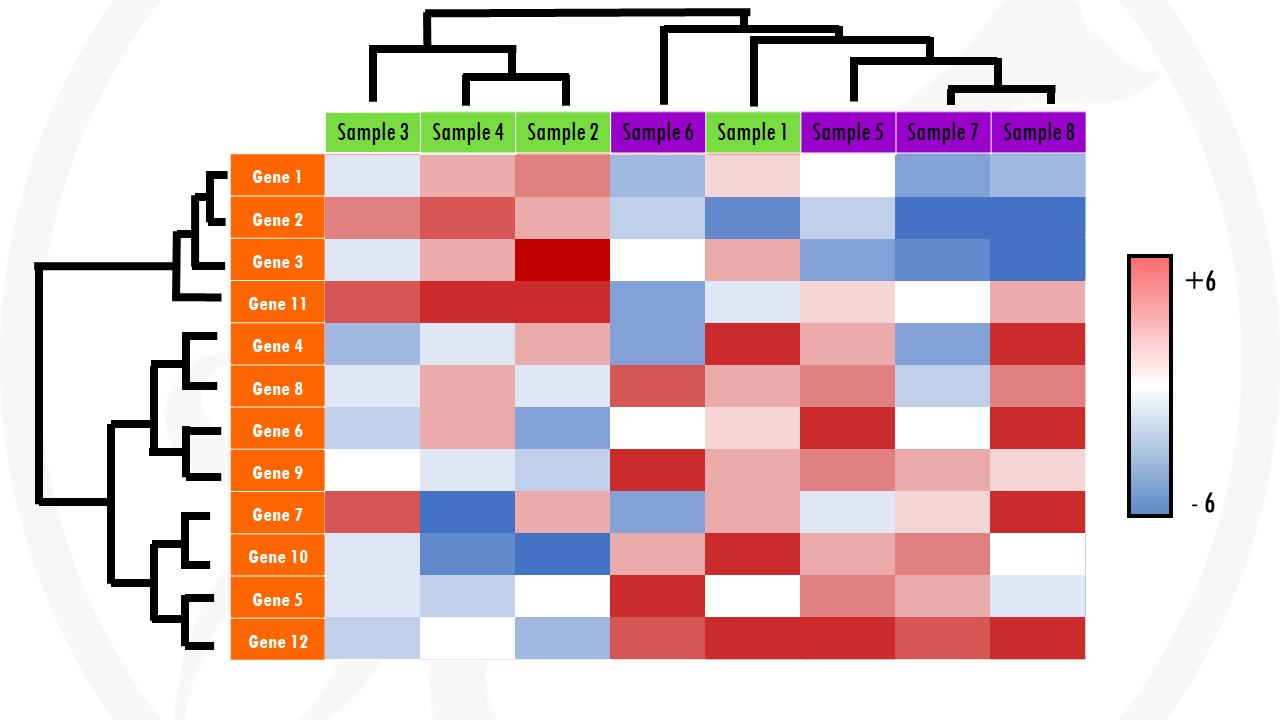
Cool right? The dendograms on the sides just indicate the results of clustering both genes and samples.
Clustered heatmaps are just heatmaps that are combined with clustering methods. This just means we group the samples and/or genes together based on the similarity of their gene expression pattern. This way, we can quickly identify samples that are more similar to each other based on their overall gene expression patterns. And we can also identify genes that have the same expression profile across all, or a certain subset of samples.
Going back to our example, a clustering algorithm will group genes with a similar expression across samples together: genes that are upregulated in all or most cancer samples, and downregulated in most or all healthy samples) will be clustered together. In a similar way, samples with a similar expression pattern (in general having certain sets of genes upregulated and certain sets of genes downregulated) will be clustered together. In our example, we expect cancer samples to cluster together, and healthy samples to cluster together… but clustering might show us unexpected (and interesting!) groupings.
In summary, adding clustering to our heatmap can be useful for identifying genes that are commonly regulated, or biological signatures associated with a particular condition (for example, a disease).
How to interpret a heatmap
There are very fancy heatmaps out there, which sometimes makes them a bit overwhelming to interpret. But don’t worry! Interpreting a heatmap is very easy.
If you would like to see a guided interpretation of a heatmap with a real-life example, check out my video! Or keep reading for a set of general guidelines to help you interpreting heatmaps.

Here are some tips to understanding any heatmap:
Check the x axis. In general, every column of the heatmap represents a different sample (cells, patients…). There should be labels – this will give you an idea of which samples are more similar to each other.
Check the y axis. In general, here you will find the genes. In cases were the heatmaps display gene expression data of hundreds of genes, the gene names may not be displayed. But it is still informative! You just have to imagine that every row of the heatmap shows the expression of a particular gene across the samples.
Check colour scale. Usually, the log2 fold change for each gene will be shown. This means that gene expression values with a log2FC > 0 will be upregulated and log2FC < 0 will be downregulated. But you do not need to worry about the actual values – check the colours! For example, upregulated genes might be in green, and downregulated genes might be displayed in red (terrible, terrible choice of colours for your colour-blind audience by the way!). Then, with a quick glance you can see patterns of upregulated (generally green areas) and downregulated genes (generally red areas).
Finally, can you identify any interesting patterns? Are there certain gene sets with differential gene expression that stand out? For example, groups of genes upregulated or downregulated in certain samples? Are there any samples that are clustered together – especially samples you didn’t expect to cluster together?
Squidtip
By the way, in this post I mainly talk about differential gene expression results, but you can also use heatmaps to display your pathway enrichment analysis results. In that case, each row is a different biological pathway.
And that is all for today! At least, concerning heatmaps. Squidtastic:)
Did you find this post useful? Is there any more topics you would like to see here?
Let me know what you think in the comments down below!
Hey, thanks very much. I wish I could get in touch.
Hi! Of course you can get in touch – you can send me a message through the Contacts page:)
Very interesting and understandable. Thank you for your effort. Actually we need also tutorial on PCA and OPLS-DA.
hi,can i discuss my data with you ? how can i get in touch
Hi! For sure, just write me an email https://biostatsquid.com/contact/